Low-resource Languages in AI Translation – A Guide for Businesses
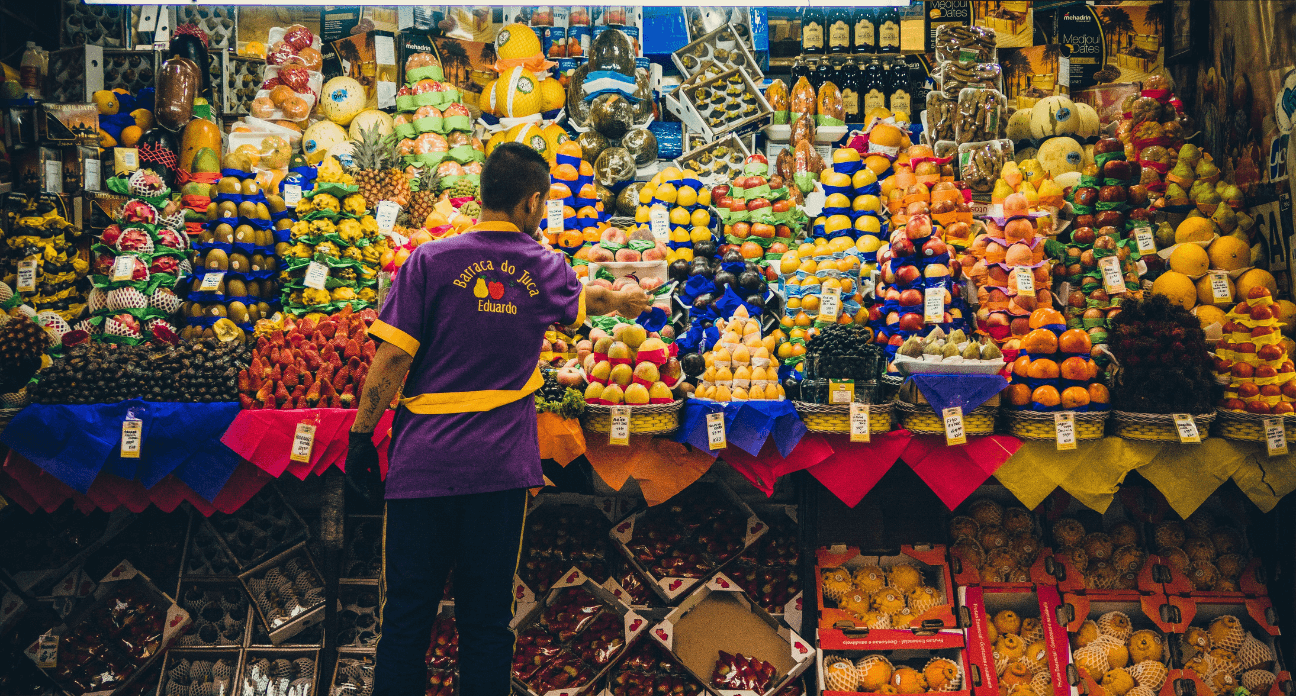
Could artificial intelligence (AI) translate Croatian proverbs in the future? Or Icelandic legalese? Irish diplomatic correspondence even?
Yes, if the PRINCIPLE Project has anything to say about it. The EU-funded initiative is backed by a consortium of research institutes and tech players who are looking to create high-quality machine translation (MT) for Croatian, Icelandic, Norwegian, and Irish. The end goal is to make having critical documents translated in the public and private sectors even faster and cheaper.
This reflects an overall trend – growing numbers of “rare” languages are being featured in translation services such as Google Translate, with the quality of the output improving by the day. Does that mean we’ll soon have the legendary Babel fish from A Hitchhiker’s Guide to the Galaxy – a tiny helper that sits in your ear and spontaneously interprets from any language in the universe? In this blog post, we’ll outline what’s currently possible with AI translation, and what belongs in the realm of science fiction (for now).
High-resource vs. low-resource languages
Artificial intelligence forms the cornerstone of modern machine translation systems. The “fuel” needed to power these engines is data. For MT systems, this is typically enormous bilingual corpora. It takes at least 10 million sentence pairs to train a machine of this nature. MT engines tend to find this training material by combing the web.
That’s why languages that have a strong Internet presence, such as English and German, are perfect for this process. These are called high-resource languages. In contrast, low-resource languages refer to languages in which significantly less content is available online. Examples include Finnish, Slovenian, and Hindi.
Yes, that’s right – Hindi! Although it’s one of the most spoken languages in the world, many official publications and commercial documents in India are available primarily in English, the country’s second official language. That’s why you’ll find comparably less high-quality bilingual content with Hindi as the source or target language on the Internet.
Is AI making low-resource languages more visible?
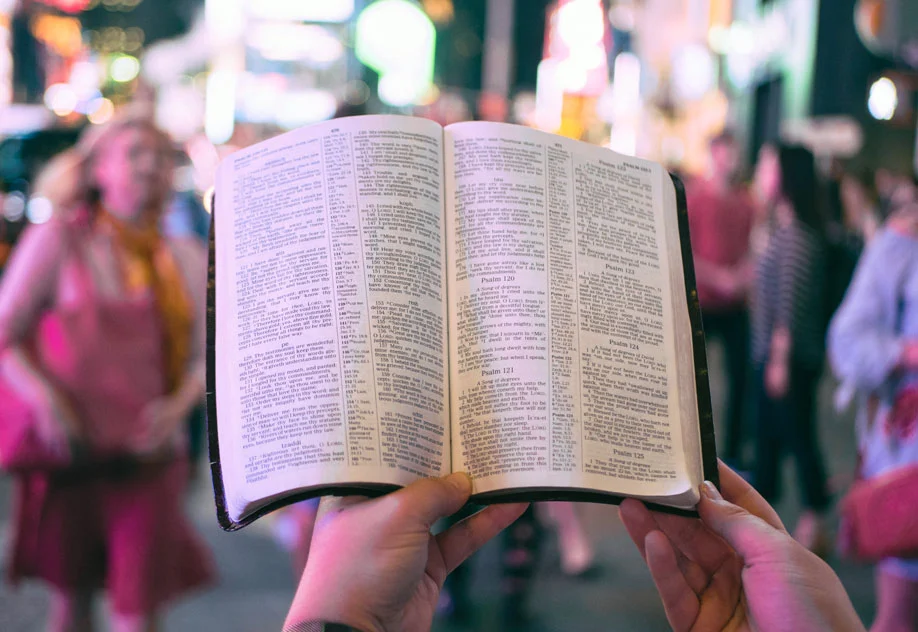
Absolutely! There are several reasons for this:
Technological architecture
Contemporary machine translation relies on neural networks and deep learning. In contrast to previous MT algorithms, this technology even works well with language combinations that have totally different grammatical structures. Machine translations from Chinese into German, for instance, are achieving a level of quality that would’ve been inconceivable a decade ago.
High maturity level of machine translation
Machine translation has reached the peak of its innovation curve. The finetuning of translation quality has slowed down for high-resource languages, shifting the focus to improving and investing in low-resource languages. This development has also seen the market diversify. While major players such as Google, Microsoft, Amazon, and IBM dominated the industry initially, more and more specialist providers that focus on one specific content type (such as medical translations) or less widespread languages have popped up in the meantime. For example, Yandex is considered an expert for Russian, Baidu has become the first port of call for Chinese, and Naver Papago is particularly popular in Korea right now.
Google has also developed a novel approach to improving the translation quality of low-resource languages through its Massively Multilingual Neural Machine Translation The word “massive” isn’t an exaggeration here – a breathtaking 25 billion sentence pairs have been fed into Google’s MT system. The solution doesn’t just cover one individual language pair – it currently supports several dozen languages and even more language combinations. Its major advantage is that the language model developed for high-resource languages can be applied to low-resource languages and used as a reference for less common language combinations, such as from French into Irish.
The best and the worst languages for machine translation
MT performs best with…
Translation into English: Translations into English are the bread and butter of many AI translation systems, as confirmed by industry reports such as Intento’s State of Machine Translation.
English ↔ Western European languages (French, German, Spanish, and so on): The vast amount of data resulting from the close political and economic ties between Western European countries significantly boosts the quality of MT in these language combinations.
Between Romance languages (French, Italian, Portuguese, Spanish): The Romance languages are united by their Latin roots. Their similar vocabulary and grammar mean machine translation works well between these languages.
Between Scandinavian languages (Danish, Norwegian, Swedish, and so on): This is another language family that is closely related, resulting in relatively accurate translations from one to another.
…and performs worst with
East Asian languages (Chinese, Japanese, Korean, and so on): Translation quality for these languages has vastly improved in recent years, but the difference between European and East Asian languages in terms of grammar, syntax, and writing systems remains a stumbling block.
African languages: The African continent has so far been severely underrepresented in research on natural language processing. We can but hope that languages which are less commercially visible or even at risk of disappearing altogether will also be given the consideration they deserve in translation research in the future.
Hungarian: Hungarian is what’s known as an agglutinative language. This means that the meaning of words and their relationship to each other are expressed by attaching sound elements (called “affixes”) to the original word. Machine translation engines struggle to interpret these major morphological changes.
Ukrainian: This is another language where Intento’s State of Machine Translation Report points out that there aren’t many translation resources available despite its large number of speakers.
… and many more!
Tip: Using machine translation for the languages above might come with a degree of risk attached – especially when dealing with sensitive content. What’s more, the translation quality will vary greatly depending on the subject area (for instance, medicine, law, journalism, everyday language). That’s why you should seek advice from a translation agency such as Milengo to pick a provider that’s right for you.
Book a free consultation call today
The future of low-resource languages
MT systems are typically trained using bilingual corpora – that is, a vast number of high-quality translations. This data is only available in fragments for low-resource languages. But are there other options?
Yes, there are. In 2017, the Transformer architecture revolutionized natural language processing and opened the door to new data sources for the training of MT systems:
- Monolingual data: Data in a single language is easier and cheaper to obtain than validated translations. These texts can contain valuable information about grammatical structures and contextual relationships.
- Auxiliary languages: These are languages that share similar syntax and semantics with the reference language but have far more linguistic resources available. Auxiliary languages can make a valuable contribution to the training of NMT models.
- Multimodal data: One experimental approach is to use audio recordings for language combinations that are very similar when spoken but differ significantly in how they’re written (such as Tajik and Persian).
- Bilingual dictionaries: Linking bilingual glossaries with monolingual data can also improve the translation of low-resource languages.
Summary
Technology has always managed to eliminate language barriers, and that’s truer now than ever before. Which is great news for companies who cater to markets in Eastern Europe, Scandinavia, Asia, and Africa. This is where machine translation will increasingly help to drive down localization costs in the future.
The market for machine translation technology is rapidly changing, and the solutions on offer tend to vary drastically in terms of quality and costs. If you want to guarantee success, then your best option is to consult an experienced translation agency such as Milengo.